Revolutionizing the Energy and Resources Industry: How AI is Reshaping the Future of Sustainability.
The energy sector has never been sceptical in adopting new technologies in the past. Be it digitization of the power generating infrastructure, exploring renewable sources of energy or innovating the aged infrastructure of transmission and distribution, it has always embraced these challenges and adopted well. However, challenges such as high carbon emissions, inefficient energy management, aging infrastructure, etc still needs to be addressed to build a more sustainable future. These challenges can be dealt with innovation and collaboration amongst stakeholders from different domains.
Artificial intelligence (AI) has the potential to transform the energy sector as it can address a wide range of problems that the sector is currently facing. The industry has already started to embrace the power of Artificial Intelligence (AI) to drive efficiency, reduce costs, and minimize carbon emissions.
According to Precedence Research, the global artificial intelligence (AI) in renewable energy market size is projected to surpass US$ 75.82 billion by 2030 and is expanding at a CAGR of 27.9% from 2021 to 2030.
In this article, we will explore some of the ways in which AI can help address the challenges that the energy sector is facing. Overcoming these challenges can create a more sustainable future for all. But before that, let's have a quick look at some of the key challenges in the energy sector.
1. Centralized power generation – majority of the global grid network relies on a few power generators making the entire infrastructure highly centralized. Though we have done a great job building such a great infrastructure, large, centralized networks have their own challenges. Some of these are high loss of energy from generation to distribution, high carbon emissions as most of these power plants are based out of non-renewable sources of energy, low power conversion rate, etc.
2. Challenges while transitioning to renewable sources – as the share of renewable sources of energy increases at a fast pace, integrating it with the existing grid system without destabilizing the grid, is quite challenging. Not only that, maintaining balance of demand and supply gets even more challenging as outputs of renewable sources of energy depends on external factors such as weather.
3. Energy Loss and theft: Improving energy efficiency is a key challenge for the energy sector. There is a need to reduce energy waste and increase the use of renewable energy sources to meet the growing demand. Theft and unethical usage of power is again a key concern causing losses to the power suppliers.
4. Ageing infrastructure: Much of the energy sector's infrastructure, such as power grids, equipment and pipelines, is ageing and in need of upgrades. This can be costly and can also create reliability issues.
Now, let’s see how AI can be leveraged to address these challenges in the energy sector.
• Renewable energy forecasting: As the share of renewable energy is increasing at a fast pace, integrating it with the existing network is challenging. One of the key reasons is the fact that the production of energy from these sources is not consistent (solar and wind power plants, their power output depends upon external weather factors). Because of this, often the forecasts are inaccurate leading to either blackouts or wastage of surplus energy. Hence, accurately forecasting the output of wind, solar, and other renewable energy sources is essential for managing energy supply and demand. AI algorithms can be used to analyse historical data and real-time weather patterns to predict renewable energy output with greater accuracy, allowing grid operators to balance supply and demand more effectively.
• Demand forecasting: AI algorithms can be used to forecast energy demand in real-time, helping grid operators to manage supply more efficiently and prevent blackouts. This can help balance supply and demand, reduce the need for backup power, and improve the overall reliability of the energy grid. This also helps in accurately planning purchase of power for different durations like intraday, short term, and long term. Other than demand forecasting, price forecasting is another important use case that helps in creating more informed power purchase agreements.
Let's have a look at the high-level architecture for demand forecasting using AWS services. With the help of AWS services like Forecast, Quicksight, Sagemaker, etc we can quickly build and deploy AI solutions at scale. Also, to make things easy, let’s assume that we are trying to forecast power demand for a utility.
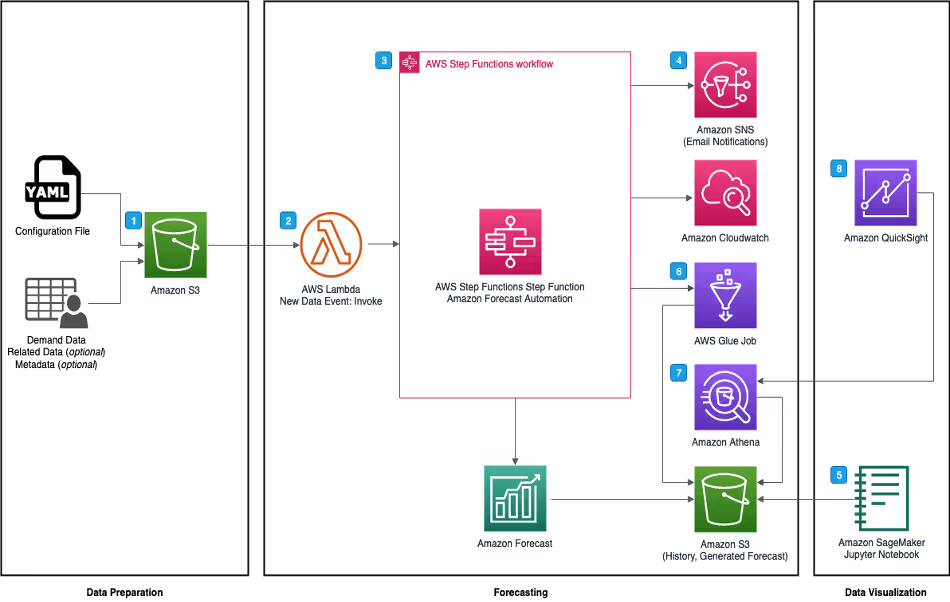
The architecture consists of four broad functional components —
• Data Preparation – First step is to prepare the demand data or the target time series, along with this we may add related time series data such as weather data, income data, and other metadata. Once this data is prepared for training, the data is uploaded to a S3 bucket from where it is used for machine learning and data visualisation tasks.
• Model Training – Once the data pre-processing is done, use AWS Forecast to create a predictor or train a custom ML model using a Jupyter notebook with SageMaker. Once the model is trained, endpoint configuration is set and the endpoint is deployed to generate forecast. Amazon CloudWatch is used to monitor the model training and its metrics and Amazon SNS (Simple Notification Service) is used to send or receive notifications for training related events.
• Forecasting/Inferencing – we can write a simple Lambda function that is triggered by S3 event notification. Whenever new data is uploaded to that S3 bucket, the Lambda function will be triggered to generate a forecast through AWS Step Function workflow which is a service to orchestrate tasks. All we need to do is upload data to the S3 bucket.
• Data Visualization – Once a forecast is generated, the results and related metrics are then saved to the s3 bucket and Amazon Athena is used to query this data. After querying the results, Amazon Quicksight is used to visualize forecast results.
• Predictive maintenance: Failure of electrical equipment leads to all sorts of undesirable conditions. These failures can lead to electrical safety hazards, unplanned downtime, increased maintenance costs, decreased equipment reliability and life span. Old predictive maintenance techniques were reliable in past but are now outdated. With reduced costs of sensors in the industry, AI solutions such as predictive maintenance can replace these old solutions and can transform entire system’s efficiency.
• Predictive maintenance uses machine learning algorithms to predict when equipment is likely to fail, based on historical data and real-time sensor readings. By identifying potential issues before they occur, maintenance teams can schedule repairs during periods of low demand, reducing downtime and costs. Also, equipment running under healthy and efficient conditions will have longer lifespan, and reduced running costs.
• Energy optimization: AWS’ collaboration with Carbon Lighthouse to reduce carbon emissions by optimizing energy usage with the help of Artificial Intelligence is a good example of energy optimization. AI solutions can be deployed to identify unoptimized scenarios and make them more efficient. Energy optimization involves using AI algorithms to optimize energy consumption across various processes, such as heating, cooling, and lighting. These algorithms learn the underlaying pattern and works to reduce power consumption by the overall process. This leads to reduced energy waste, improved efficiency, and lower costs.
• Fraud Detection: Electricity theft and fraud is not a new challenge, it has been there for a long time and fraudsters keep innovating newer methods of unaccounted power consumption. However, with the help of new technology such as AI, more robust anti-theft systems can be built to help minimize such events. AI can be used to detect fraudulent activity in the energy sector, such as meter tampering or theft of service. By analysing usage patterns and other data, AI can identify suspicious behaviour and alert utilities to potential problems, helping to reduce losses and improve overall revenue.
• Power Management – Wastage of power due to unoptimized solutions or human ignorance is a key concern when it comes to power management. When AI is integrated with sensors (IoT), we get a complete power management toolkit that can be installed anywhere from residential houses to commercial buildings. These AI powered smart solutions can help companies to cut down their utility bills by a significant amount. Till we have “arc-reactors” powering our buildings like Tony Stark’s NYC lab, we need an AI to at least manage the power of our buildings.
The energy sector is facing significant challenges in the modern world, including high centralization, transitioning to renewables, energy losses and thefts, and ageing infrastructure. To overcome these challenges, the industry must embrace innovation and collaborate with stakeholders to create sustainable solutions. AI is one such innovation that can help reduce carbon emissions, optimize energy production and distribution, manage renewable energy sources, and improve energy efficiency. By leveraging the power of AI, the energy sector can create a more sustainable future for all, while also ensuring reliable and secure energy supplies. The road ahead may be challenging, but with innovative solutions and collaborative efforts, we can build a brighter and cleaner future for generations to come.
— Author Rishi Khandelwal